
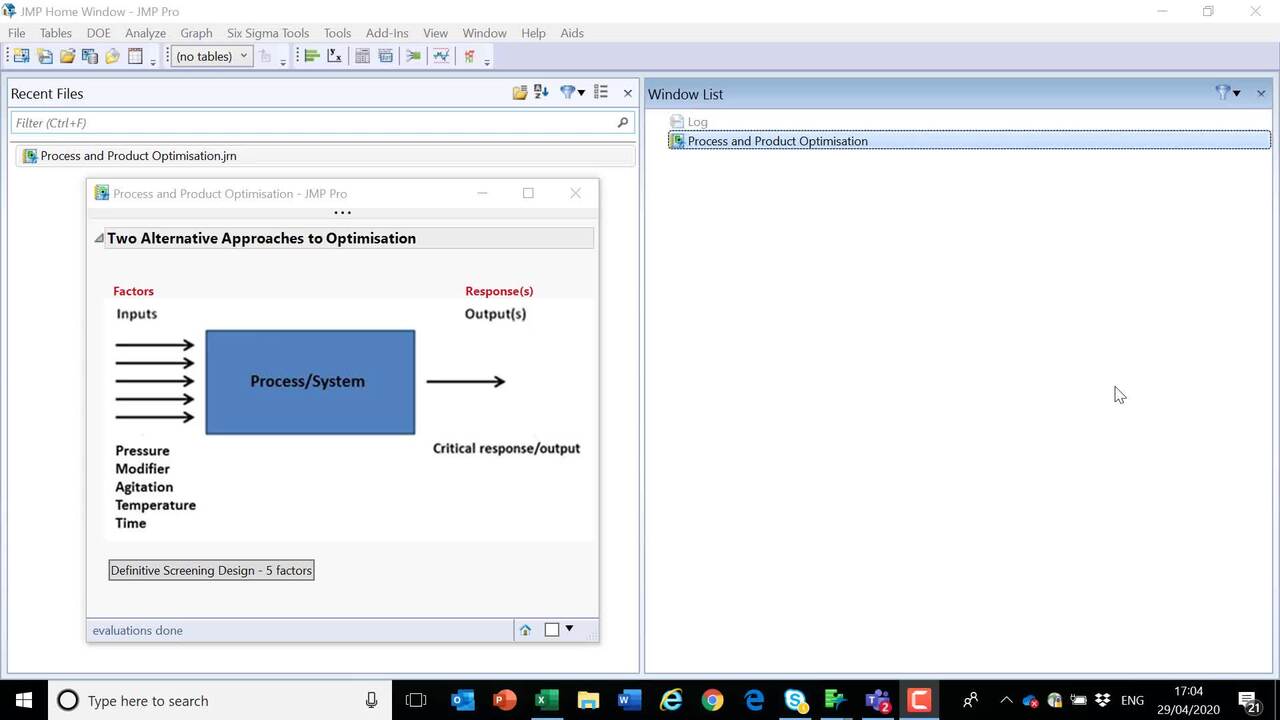
JMP offers all of the classical design types you would expect, including Full Factorial, Screening, Response Surface, Mixture and Taguchi Array.
#SIMULATE RESULTS IN JMP 13 SERIES#
Despite this burden, the ingenuity of practitioners over more than 80 years has led to a series of widely applied design families adapted to meet specific situations and experimental objectives. But until relatively recently, generating (and then analyzing) a design to exploit these principles relied primarily on hand calculation. Ronald Fisher first introduced four enduring principles of DOE: The factorial principle, randomization, replication and blocking. Once the data has been collected, JMP streamlines the analysis and model building so you can easily see the pattern of response, identify active factors and optimize responses. In addition to a complete library of tried and tested classical DOE designs, JMP also offers an innovative custom design capability that tailors your design to answer specific questions without wasting precious resources. To properly uncover how factors jointly affect the response, you need to use design of experiments (DOE). However, whenever there is more than one factor – that is, in almost all real-world situations – a design that changes just one factor at a time is inefficient. Actively manipulating factors according to a pre-specified design is the best way to gain useful, new understanding. To reveal or model relationships between an input, or factor, and an output, or response, the best approach is to deliberately change the former and see whether the latter changes, too. Methodical experimentation has many applications for efficient and effective information gathering.
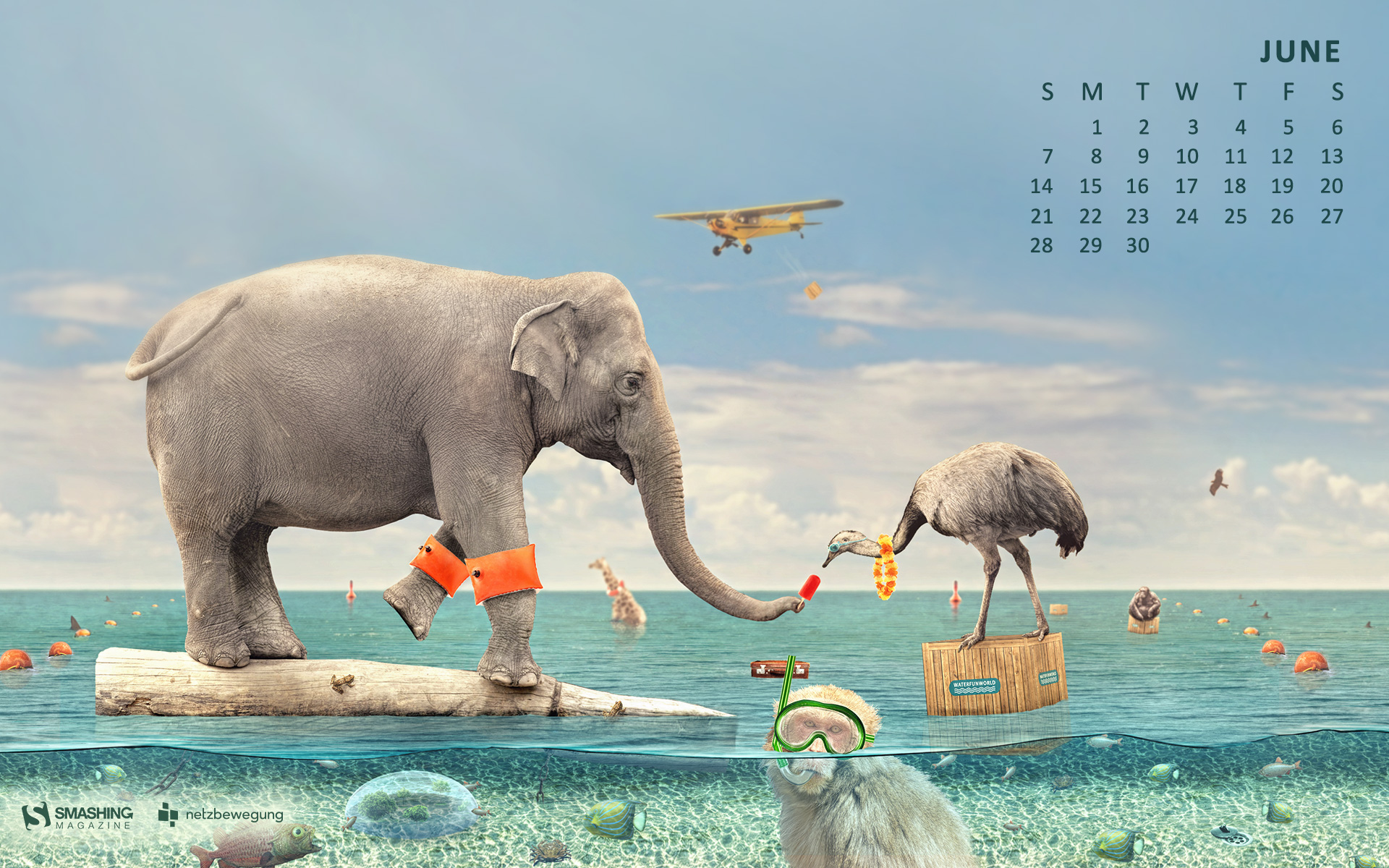
Design of experiments, or DOE, is a practical and ubiquitous approach for exploring multifactor opportunity spaces, and JMP offers world-class capabilities for design and analysis in a form you can easily use.
